by Roman Egger
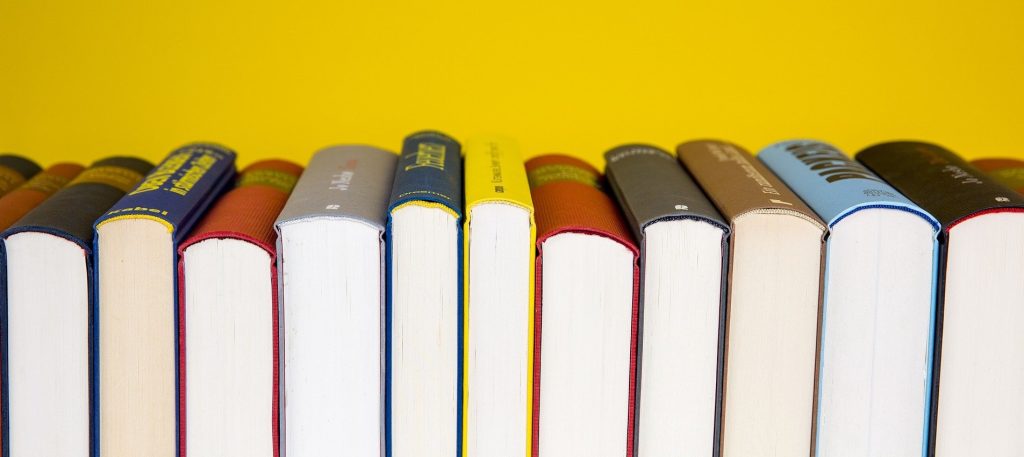
Natural Language Processing is developing rapidly and not only the performance of the algorithms is constantly increasing but also their use is becoming much easier. AI communities like Huggingface make a variety of state-of-the-art models accessible and usable with just a few lines of code. For this reason, I took a look at two pipelines and tried them out. On the one hand, I will try to generate a longer text from a short input text, in the second case the opposite should happen and a text should be summarized.
Huggingface offers the pipeline “text-generation” for the generation of text. For tasks like this, huge transformer-based language models are trained on the basis of millions of text data. Huggingface offers access to well-known models such as GPT2 from Open AI or XLNet.
For the task of text generation, I used the first paragraph of this post as input. It only takes three lines of code to try out this task. I used “max_length = 300” to define the maximum length of the generated text.

The first part of the result (here in italics) repeats the input text. Then the model tries to continue with the automatically generated text.
[{'generated_text': "Natural Language Processing is developing rapidly and not only the performance of the algorithms is constantly increasing but also their use is becoming much easier. AI communities like Huggingface make a variety of state of the art models accessible and usable with just a few lines of code. For this reason, I took a look at two pipelines and tried them out. One is to try to generate a new text from a short existing text, in the second case text summarization is to be used.\n\nThe data set was divided into two main tasks. First, it needed to get the language in the system, on a local and remote server. Second, we wanted to get a translation of our text for the system.\n\nTo do it, I ran a bot which is very simple and easy to learn. On a local and remote, the system translates the text from local to remote but in addition, the system needs some resources to communicate with the local server. The language model is a state of the art. It is designed mostly with local language recognition in mind, making it far easier to understand a native language as it changes through the code.\n\nWhen we try to find that there are problems in the code then our interpreter will interpret them in a way that will reduce the speed of our language learning. For a few lines of code, our bot creates a bot.js file in the system, in a specific file, in the server's root project directory. Now, we could try to apply"}]
How far this text makes sense is up to the reader. Of course, the input is largely responsible for the quality of the output.
For the summary of a text, the pipeline “summarization” is used.

[{'summary_text': ' AI communities like Huggingface make a variety of state of the art models accessible and usable with just a few lines of code . For this reason, I took a look at two pipelines and tried them out . One is to try to generate a new text from a short existing text, in the second case text summarization is to be used .'}]
Again, I used the first paragraph of this blog post as input, and as you can see this task can be solved quite satisfactorily.